NVIDIA is Offering its Trained Deep Neural Networks to the Industry to Accelerate the Development of Autonomous Vehicles
【Summary】Nvidia announced this week at the company’s GTC technology conference in China that it will provide the transportation industry with access to its NVIDIA DRIVE trained deep neural networks (DNNs) for autonomous vehicle development.
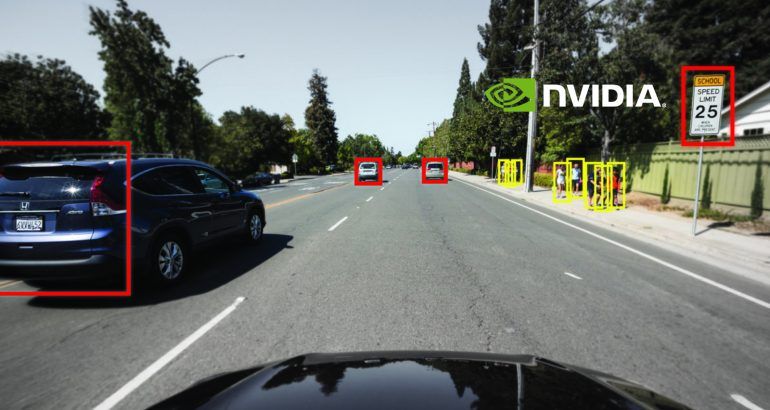
For developers using AI for autonomous driving applications, one of the biggest tasks is training deep neural networks. For self-driving vehicles, neural networks are used for complex tasks such as identifying street signs, detecting pedestrians and navigation.
However training machine learning models for these tasks requires an enormous amount of data and compute power. In addition, collecting and labeling training data it is long and painstaking process, especially for many of the budding startups working on autonomous driving.
Nvidia is making its easier and the company announced this week at the company's GTC technology conference in China that it will provide the transportation industry with access to its NVIDIA DRIVE pre-trained deep neural networks (DNNs) for autonomous vehicle development on the Nvidia GPU Cloud (NGC) container registry.
Using a suite of Nvidia AI tools, developers using the DRIVE ecosystem can freely extend and customize the models to increase the robustness and capabilities of their own self-driving systems.
AI is central to the development of safe, self-driving vehicles — allowing them to perceive and react in real time to their surroundings. A typical self-driving vehicle can use dozens of DNNs to ensuring accurate perception, localization and path planning.
Nvidia said it has spent years developing and training its DNNs designed to run on the NVIDIA DRIVE AGX platform. The DNNs are used to take vast amounts of raw sensor data and turn it into a deep understanding of the environment.
These pre-trained DNNs cover such tasks as traffic-light and road sign detection, object detection (for vehicles, pedestrians, bicycles) and path perception, as well as gaze detection and gesture recognition inside the vehicle which are used in driver monitoring systems.
"NVIDIA leads the world in developing the deepest and broadest suite of DNNs and AI tools for the transportation industry," said Luca De Ambroggi, senior research director of Artificial Intelligence at IHS Markit. "Making these algorithms available to others, along with the tools and workflow infrastructure to customize them, will help enable the deployment of safe autonomous transportation."
Nvidia's NGC (cloud) containers reduce the complexity of setting up software, so developers can focus on the problem solving aspects of their projects. By taking care of most of the work behind the scenes, access to Nvidia's container registry enables users to focus on building better models.
Nvidia is not the only tech company sharing its training data. In August, Waymo, which spun out of Google's early self-driving car project, released the" Waymo Open Dataset" for autonomous vehicle researchers.
The Waymo Open Dataset, which is available for free, covers a wide variety of environments, from dense urban centers to suburban landscapes. It also includes data collected during day and night, at dawn and dusk, in sunshine and rain. Waymo said the data was collected by a fleet of Waymo self-driving vehicles that traveled over 10 million miles in 25 different cities.
In July, Ride-hailing company Lyft released its own "Level 5 Dataset." The data is also what the ride-sharing company calls is the "largest publicly released dataset of its kind." Lyft's dataset includes over 55,000 3D frames of footage that's been captured by human reviewers. It also includes a HD spatial semantic map.
Lyft chose to release its dataset to "help level the playing field for researchers interested in autonomous technology."
"The AI autonomous vehicle is a software-defined vehicle required to operate around the world on a wide variety of datasets," said Jensen Huang, founder and CEO of NVIDIA. "By providing AV developers access to our DNNs and the advanced learning tools to optimize them for multiple datasets, we're enabling shared learning across companies and countries, while maintaining data ownership and privacy. Ultimately, we are accelerating the reality of global autonomous vehicles."
Advanced Learning Tools for AI Development
In addition to providing access to the DNNs, Nvidia announced the availability of a suite of advanced tools so developers can customize and enhance NVIDIA's DNNs using their own datasets and target feature set. These tools allow the training of DNNs using active learning, federated learning and transfer learning.
Over the past several years, Nvidia's DRIVE platform has become the go-to product for developing autonomous vehicles. It's robust processing power and software is used by automakers, tech startups and universities all around the world for autonomous vehicle development.
By providing access to its AI models on NGC and introducing advanced training tools, Nvidia is building up its end-to-end platform for autonomous vehicle development and deployment. With the release of dataset, developers are getting access to the same pre-trained DNNs that Nvidia uses to improve upon its DRIVE autonomous driving ecosystem.
-
Ford is Testing a New Robotic Charging Station to Assist Drivers of EVs With Disabilities
-
Ford Raises the Prices of the F-150 Lightning Electric Pickup Due to Rising Raw Material Costs
-
The BMW 7-Series to Feature HD Live Maps From HERE Technologies for Hands-Free Highway Driving in North America at Speeds up to 80 MPH
-
AutoX to Use the 'Eyeonic Vision Sensor' from California-based SiLC Technologies for its Robotaxi Fleet in China
-
LG Develops ‘Invisible’ Speaker Sound Technology That Could Revolutionize In-Vehicle Audio
-
Researchers at South Korea’s Chung-Ang University Develop a ‘Meta-Reinforcement’ Machine Learning Algorithm for Traffic Lights to Improve Vehicle Throughput
-
Zeekr’s New 009 Electric Passenger Van is the World’s First EV to Feature CATL’s Advanced ‘Qilin’ Battery With a Range of 510 Miles
-
Redwood Materials is Building an Electric Vehicle Battery Recycling Facility in South Carolina
- LG Chem is Investing $3.2 Billion to Build a Cathode Manufacturing Facility for Electric Vehicle Batteries in Tennessee
- Qualcomm and its Industry Partners Demonstrate C-V2X Technology in Georgia That Ensures School Buses and Fire Trucks Never Get Stuck at Red Lights
- Reservations for the Electric Fisker Ocean SUV Reach 50,000, The Company Shares New Details About its Second EV Being Built by Foxconn
- Ford Motor Co is Recalling 2.9 Million Vehicle That Could ‘Roll Away’ After the Transmission is Shifted Into Park
- Toyota and Stellantis to Partner on a Large Commercial Van for the European Market, Including an All-Electric Version
- AI-Powered Computer Vision Perception Developer StradVision Closes on $88 Million Series C Funding Round
- Tesla Believes Its Dojo AI System Will Help It Win the Self-Driving Car Race
- Researchers at South Korea’s Chung-Ang University Develop a ‘Meta-Reinforcement’ Machine Learning Algorithm for Traffic Lights to Improve Vehicle Throughput
- Audi Hits EV Startup NIO With a Trademark Lawsuit Over its Vehicle Naming
- EV Startup VinFast is Offering 3 Years of Free EV Charging and Advanced Driver Assist System for Customers That Reserve a Vehicle Through Sept 30